Apparently You Can Teach an Old Machine New Tricks
By: Austin Tucker, Sr. Underwriting Product Manager – Verisk
Do you remember learning multiplication in grade school? It was probably a challenging experience, and it may have taken you a while to figure out that dreaded multiplication table. Yet, you didn’t fret because the teachers gave tools to help you learn this new skill. While some teachers taught skip counting or to find patterns in the numbers, we were all given flashcards.
Even the words flashcards can send chills up the spine of some adults to this day.
We sat there for an eternity flipping through these cards with a multiplication problem on the front and the answer on the back. Guess, look at the back, see if you were right, and divide into a pile of “got right” and “got wrong” and then go back through the wrong stack till it joined its brethren in a single mini tower of frustration.
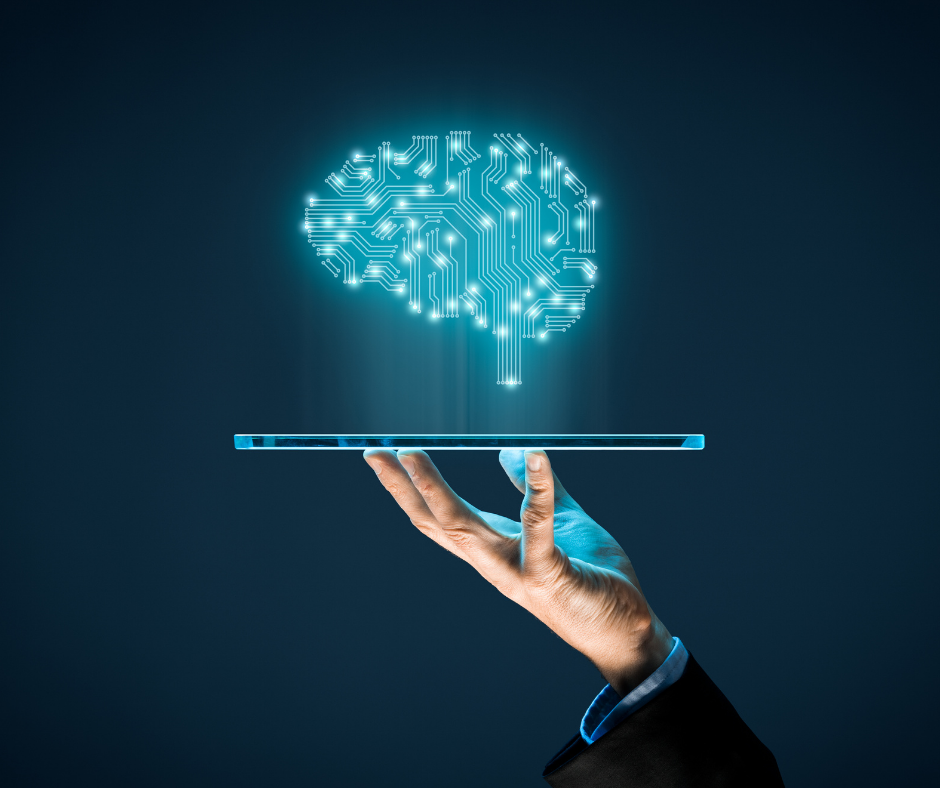
For machine learning (ML), labeled examples are their version of flashcards. The standard technique teaches a machine to accurately recognize an object, picture, video, words, or any other sort of information you must provide labeled data to the machine until it can make better predictions. The more data feed into the machine, the better the prediction.
While this method works and has proven itself, it takes a significant toll on resources of computational time, staff cost, funding, and other resources that have made it impracticable on a large scale. A company can deploy a method called few-shot (FSL) learning can be used to reduce the burden on resources as well as create more robust predictions.
What makes FSL so important to ML is that it not only reduces the effort of data collection, data labeling, and computational costs but it also allows the computer to learn about rare cases (the computer can classify, i.e., a rare animal species that is rarely photographed with only a handful of prior information). The best (and most frightening) way to describe FSL’s capabilities is to say that it can learn like a human. Humans can spot differences in a data set (i.e., handwriting, pictures of dogs) with only a few examples, whereas the traditional ML approach required large amounts of data to spot any differences; with FSL, this learning time is reduced drastically, and a machine can learn from only a few examples to spot discrepancies.
FSL is considered a meta-learning model, which means the model learns how to learn to solve a given problem or “learning the learner.” This method improves a model’s results by changing the learning algorithm via experimental results. This meta-learning model helps researchers know which algorithms make the best predictions in a dataset.
FSL can be found in several applications that solve problems:
- Computer vision- image classification, character recognition, object tracking, and others
- Natural Language Processing- sentence completion (Gmail and Outlook email), text classification, and translations
- Audio Processing- voice cloning (Siri, Alexa, GPS voices, etc.), voice conversions across different languages
- Robotics- movement learning, visual navigation, etc.
- Healthcare- drug discovery, disease diagnosis
- IOT analytics and mathematical reasoning
This may seem a bit out of scope for insurance but consider the rise in smartphone apps that have taken over a role that was once a job for someone in the insurance space. We can now use our phones to take photos of the damage to our homes, cars, places of business and upload them to our insurance carrier’s claim app to start the claims process or even settle some claims in seconds. Trying to teach these ML models to spot all kinds of damages, verify the integrity of the photos, and make essential decisions would be an IT lift that would stagger most companies.
The advent of FSL has helped shorten the time to market for these capabilities and will continue to do so newer models such as one-shot and no-shot learning comes more common. While the increasing complexity of the insurance world becomes more and more digitized, the applications for these and other ML and artificial intelligence-based assistants will continue to grow to previously unimaginable heights in insurance and our lives.
Leave a Reply
Want to join the discussion?Feel free to contribute!